Caution
You're reading an old version of this documentation. If you want up-to-date information, please have a look at 0.9.1.
Note
Click here to download the full example code
Superflux onsets¶
This notebook demonstrates how to recover the Superflux onset detection algorithm of Boeck and Widmer, 2013 from librosa.
This algorithm improves onset detection accuracy in the presence of vibrato.
# Code source: Brian McFee
# License: ISC
We’ll need numpy and matplotlib for this example
from __future__ import print_function
import numpy as np
import matplotlib.pyplot as plt
import librosa
import librosa.display
We’ll load in a five-second clip of a track that has noticeable vocal vibrato. The method works fine for longer signals, but the results are harder to visualize.
Out:
/tmp/tmpfl4ra6qp/b0064fe7dbe8048b1d4148e61a568b6fe3fca91b/librosa/core/audio.py:161: UserWarning: PySoundFile failed. Trying audioread instead.
warnings.warn('PySoundFile failed. Trying audioread instead.')
These parameters are taken directly from the paper
The paper uses a log-frequency representation, but for simplicity, we’ll use a Mel spectrogram instead.
S = librosa.feature.melspectrogram(y, sr=sr, n_fft=n_fft,
hop_length=hop_length,
fmin=fmin,
fmax=fmax,
n_mels=n_mels)
plt.figure(figsize=(6, 4))
librosa.display.specshow(librosa.power_to_db(S, ref=np.max),
y_axis='mel', x_axis='time', sr=sr,
hop_length=hop_length, fmin=fmin, fmax=fmax)
plt.tight_layout()
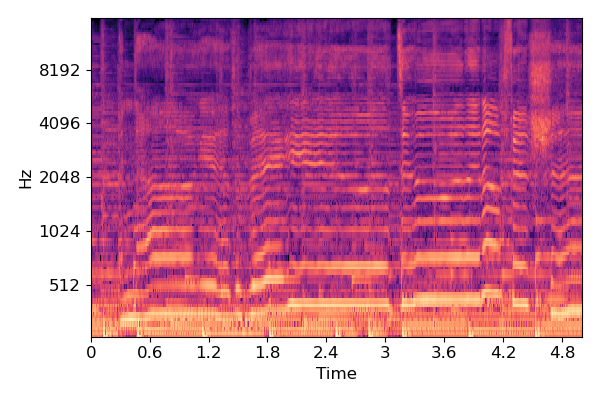
Out:
/tmp/tmpfl4ra6qp/b0064fe7dbe8048b1d4148e61a568b6fe3fca91b/librosa/display.py:862: MatplotlibDeprecationWarning: The 'basey' parameter of __init__() has been renamed 'base' since Matplotlib 3.3; support for the old name will be dropped two minor releases later.
scaler(mode, **kwargs)
/tmp/tmpfl4ra6qp/b0064fe7dbe8048b1d4148e61a568b6fe3fca91b/librosa/display.py:862: MatplotlibDeprecationWarning: The 'linthreshy' parameter of __init__() has been renamed 'linthresh' since Matplotlib 3.3; support for the old name will be dropped two minor releases later.
scaler(mode, **kwargs)
Now we’ll compute the onset strength envelope and onset events using the librosa defaults.
odf_default = librosa.onset.onset_strength(y=y, sr=sr, hop_length=hop_length)
onset_default = librosa.onset.onset_detect(y=y, sr=sr, hop_length=hop_length,
units='time')
And similarly with the superflux method
odf_sf = librosa.onset.onset_strength(S=librosa.power_to_db(S, ref=np.max),
sr=sr,
hop_length=hop_length,
lag=lag, max_size=max_size)
onset_sf = librosa.onset.onset_detect(onset_envelope=odf_sf,
sr=sr,
hop_length=hop_length,
units='time')
If you look carefully, the default onset detector (top sub-plot) has several false positives in high-vibrato regions, eg around 0.62s or 1.80s.
The superflux method (middle plot) is less susceptible to vibrato, and does not detect onset events at those points.
# sphinx_gallery_thumbnail_number = 2
plt.figure(figsize=(6, 6))
frame_time = librosa.frames_to_time(np.arange(len(odf_default)),
sr=sr,
hop_length=hop_length)
ax = plt.subplot(2, 1, 2)
librosa.display.specshow(librosa.power_to_db(S, ref=np.max),
y_axis='mel', x_axis='time', sr=sr,
hop_length=hop_length, fmin=fmin, fmax=fmax)
plt.xlim([0, 5.0])
plt.axis('tight')
plt.subplot(4, 1, 1, sharex=ax)
plt.plot(frame_time, odf_default, label='Spectral flux')
plt.vlines(onset_default, 0, odf_default.max(), label='Onsets')
plt.xlim([0, 5.0])
plt.legend()
plt.subplot(4, 1, 2, sharex=ax)
plt.plot(frame_time, odf_sf, color='g', label='Superflux')
plt.vlines(onset_sf, 0, odf_sf.max(), label='Onsets')
plt.xlim([0, 5.0])
plt.legend()
plt.tight_layout()
plt.show()
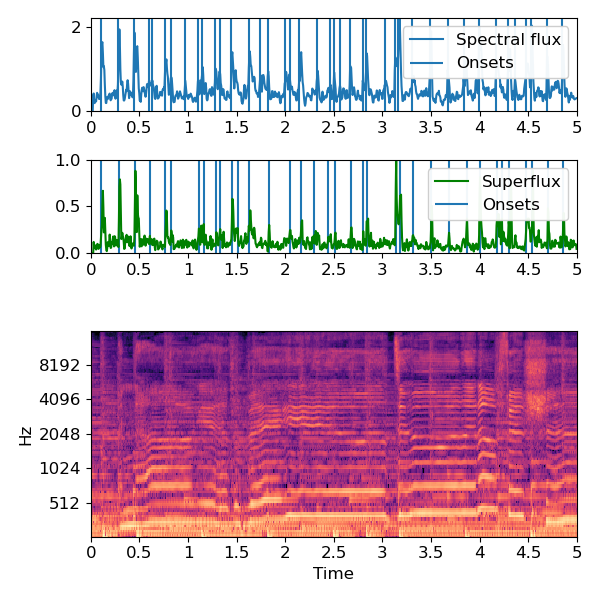
Out:
/tmp/tmpfl4ra6qp/b0064fe7dbe8048b1d4148e61a568b6fe3fca91b/librosa/display.py:862: MatplotlibDeprecationWarning: The 'basey' parameter of __init__() has been renamed 'base' since Matplotlib 3.3; support for the old name will be dropped two minor releases later.
scaler(mode, **kwargs)
/tmp/tmpfl4ra6qp/b0064fe7dbe8048b1d4148e61a568b6fe3fca91b/librosa/display.py:862: MatplotlibDeprecationWarning: The 'linthreshy' parameter of __init__() has been renamed 'linthresh' since Matplotlib 3.3; support for the old name will be dropped two minor releases later.
scaler(mode, **kwargs)
Total running time of the script: ( 0 minutes 0.691 seconds)